AI for Population Health Management
October 2024
Well Solutions Group
AI Practical Uses and Understanding
STARTING WITH THE FUNDAMENTALS
This is the first in our short series of articles about prospects for advancements in population health management (and health care overall) through the application of Artificial Intelligence (AI). Here we set out to provide a general overview and orientation on this topic, to be followed with subsequent article(s) delving further into how to get started with AI and suggested applications for practical and effective use.
Vivamus magna justo, lacinia eget consectetur sed, convallis at tellus. Lorem ipsum dolor sit amet, consectetur adipiscing elit. Quisque velit nisi, pretium ut lacinia in, elementum id enim. Vivamus magna justo, lacinia eget consectetur
%
healthcare AI Growth Rate
%
healthcare industry adoption
%
AI used for diagnosis
Why Artificial Intelligence (AI)?
We are fast approaching a new reality from “Alexa, play Bohemian Rhapsody” to “Alexa, what is the best medical treatment plan for me?”
Have you asked Siri or Alexa to play your favorite song today? Well, it is not inconceivable that in the not-too-distant future, AI may help physicians identify patient populations at risk for illness or an adverse health event (like a heart attack or stroke) with much greater precision. AI has come a very long way, and its future potential in all aspects of healthcare from prevention and diagnosis, through treatment, is nothing short of immense. AI has the potential to reimagine healthcare through technologies and data applications that can anticipate, interpret, and learn in the same ways as human intelligence.
Feeling a bit skeptical?
If so, you are not alone. However, having some doubts should not stand in the way of exploring the possibilities, as even the more transactional applications of AI are optimized via ongoing experimentation and learning. Based upon our own initial forays into the world of AI, we often encourage our clients to begin by imagining the possible – while thinking big but acting small. Start with a practical application of AI that has a tangible expected benefit – something you can measure, validate, and get comfortable with.
AI – Not the Same Hype as “Big Data”
Speaking of thinking big, enthusiasm surrounding the potential of AI has been tempered of late by expectations that it will entail the same level of financial and other resource demands as “Big Data.” Just a few short years ago, all the hype was about Big Data, however health care organizations quickly discovered that diving into the deep end of the Big Data pool involved a sizable commitment of time, money, and resources. Fast forward to today where all the talk has shifted to AI. Given the prior experience with Big Data, many organizations are cautious about taking the plunge, wary of having to make big investments to even get started.
Let’s look at the differences between AI and Big Data to gain some useful perspective about how the two are different, in this regard:
“Start with a practical application of AI that has a tangible expected benefit – something you can measure, validate, and get comfortable with.”
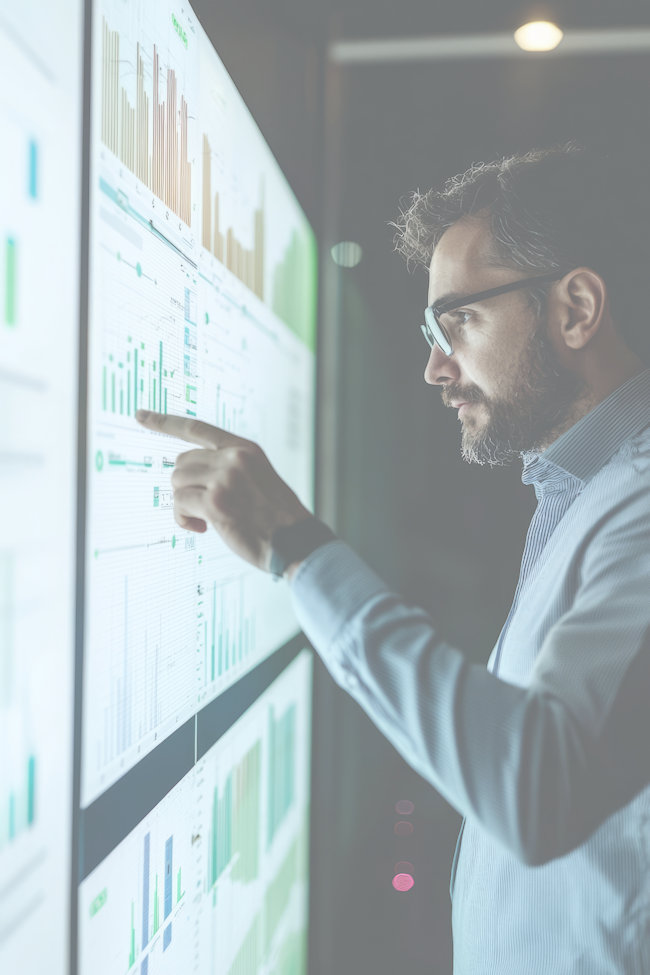
The difference between AI and Big Data lies in the utility and result of each
AI analyzes data inputs to learn and improve sorting and/or patterning of information over time, using data gathered and available along the way to provide a more accurate result. In contrast, Big Data is the overarching pool of information that is accumulated from various data sources, thus enabling but fulfilling a very different role than AI.
Big Data is all about managing large amounts of data from a variety of sources. It comes into play when the volume of data is too large for traditional data management practices to be effective. Organizations have long collected massive amounts of information about the people they serve and the services they provide, but eventually the volume of data proves too unwieldy and the return on investment from these growing and diverse data quickly diminishes. This is where Big Data comes into play. It is about collecting, organizing, and storing diverse data assets so that it can be more efficiently and effectively put to use.
There is however a fairly heavy resource commitment associated with Big Data. It starts with access to and investment in the data itself to get it pulled together in a usable way via data quality, data formatting and wrangling, all the way through to establishing the infrastructure and organization required to connect all of the elements prior to deriving any value.
One of the biggest reasons that an investment in Big Data fails to pay off is that many organizations haven’t done a good job with the information they already have. They have not fully leveraged the existing data in ways that enhance their understanding and then make changes in response to new insights. Those competencies don’t just materialize because organizations have invested in high-end data.
Artificial Intelligence (AI) refers to technologies which enable computers to simulate human intelligence, capable of logic, reasoning, and decision-making. This “self-learning” technology uses detection of patterns to analyze data and then outputs actionable insights in a more efficient manner than human-driven methods. AI can identify data types and find new connections and/or patterns among various data points. It can be used to automate and accelerate data preparation tasks, including the generation of data models, and assist in data exploration – even detecting and resolving data discrepancies and/or inconsistencies.
There is clearly a synergistic relationship between Big Data and AI. In an ideal end state, Big Data provides the fuel that powers the evolution of AI decision making, while AI assists in all phases of Big Data and processes involved in its aggregation, storage, and retrieval of diverse types of data from various sources.
In other words, in today’s world, deriving value from Big Data benefits from already having basic AI capabilities in place to get the data in shape for maximum value. For organizations that have already made the investment, Big Data provides the raw material, but AI enables generation of the higher-level insights that make the investment in Big Data worth it.
“One of the biggest reasons that an investment in Big Data fails to pay off is that many organizations haven’t done a good job with the information they already have.”
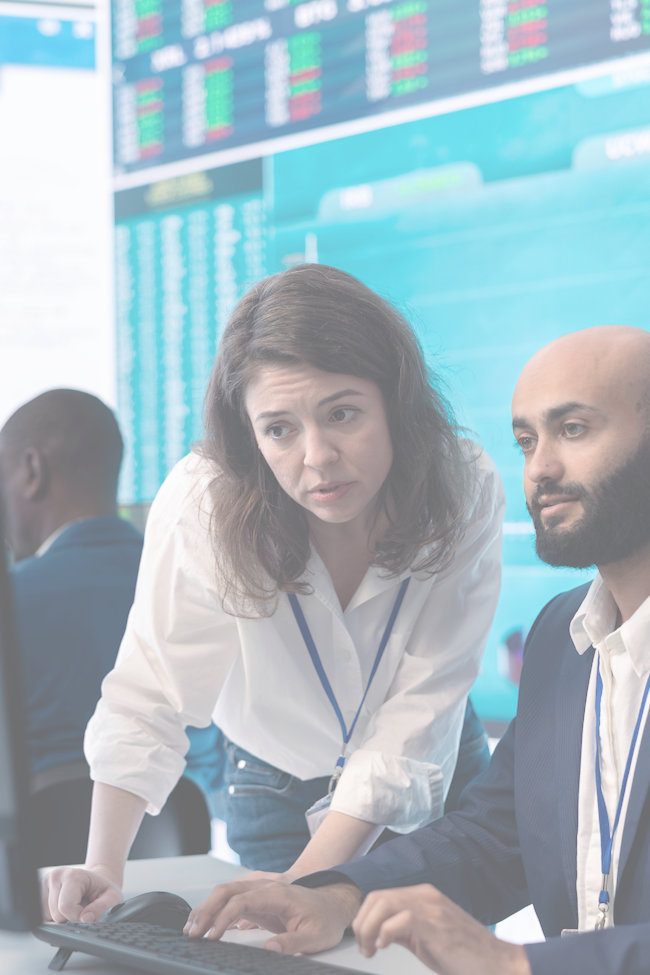
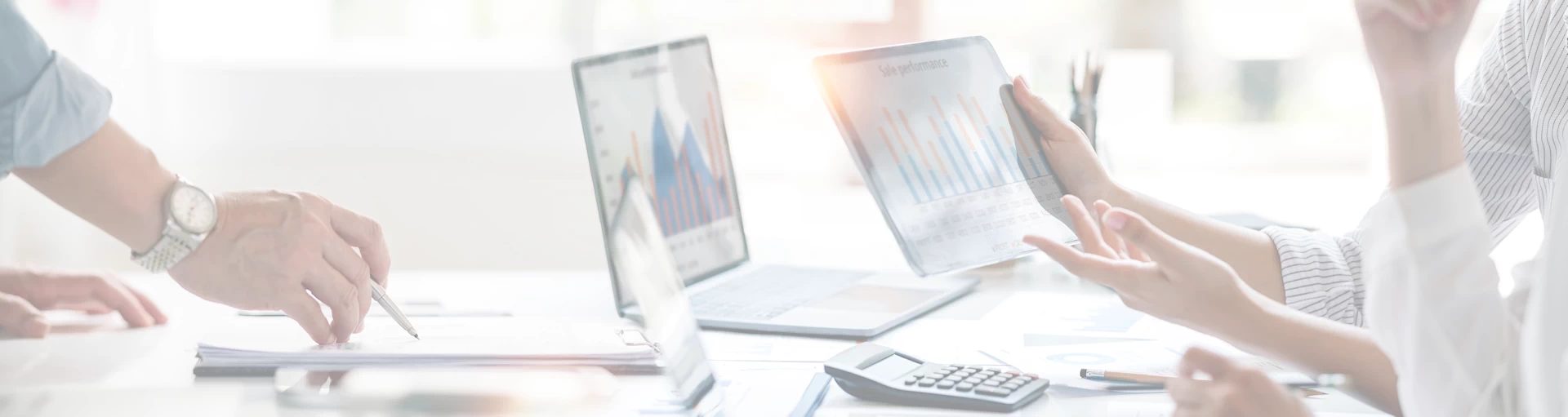
The good news, however, is that there are ways in which organizations can leverage AI to add value, with or without Big Data.
Thinking of starting to explore where AI can play a role?
Machine learning, one of the cornerstones of AI applications, provides considerable value via data driven algorithms and statistical models that can analyze and find patterns in data. This is different from traditional rules-based approaches that follow explicit instructions.
Digital footprints are growing at a rapid pace and forward-looking health care organizations are seeking to use this to their advantage via greater insights into each individual. Rather than manually moving data in and out of warehouses and creating static reports, AI can provide automated and intelligent analytic tools that access available data from disparate sources, generating fresh insights directly from the sum of the data available at any point in time.
This can be done without making major investments in Big Data.
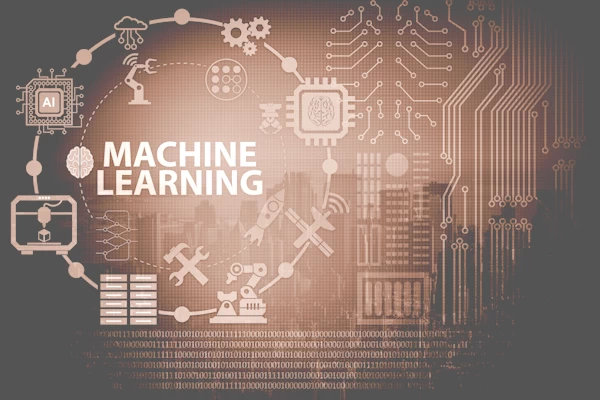
Continuous Learning
In the past, organizations built complex, rules-based systems for a wide range of data reporting needs but found these solutions too static to handle rapidly changing data and circumstances.
Now with the power of AI, companies are building systems that “learn” from the continual influx of new data. This improves decision making, business intelligence and predictive analytics.
Put AI to Use: Real-World Examples
- Improve acquisition and retention – Organizations can derive a better handle on what their patients and/or customers are interested in, how products and services are being used and reasons why people engage or disengage in clinical and health management programs and services. There is a growing opportunity to leverage consumer data that exists outside of healthcare to develop insights and strategies for segmentation of populations in order to pinpoint new areas of opportunity as well as existing product and services enhancements.
- Predict and forecast demand – Instead of basing estimates of current year’s enrollment and use of health programs on data from the prior year, AI can be set up to scan a variety of factors such as changing practices in health care, the advent of a global pandemic, and other hard to predict factors, in order to spot patterns and trends early and know how they may impact future performance of population health management programs.
- Cybersecurity and fraud prevention – Organizations are using AI powered analytics to identify patterns of fraud and abuse via detection of anomalies in the data, enabling the ability to sort through a variety of transactions to not only identify and detect but also to prevent and mitigate potential fraudulent behavior.
The key takeaway here is that, while the benefits of Big Data and AI combined yield demonstrable power and utility, organizations can absolutely start small and begin to apply AI to the data that is currently available. They can begin to derive new insights, without making major investments in data stores and data systems out of the gate. AI does not necessarily involve a major investment up front – in fact, it can make the data you already have better and more insightful.
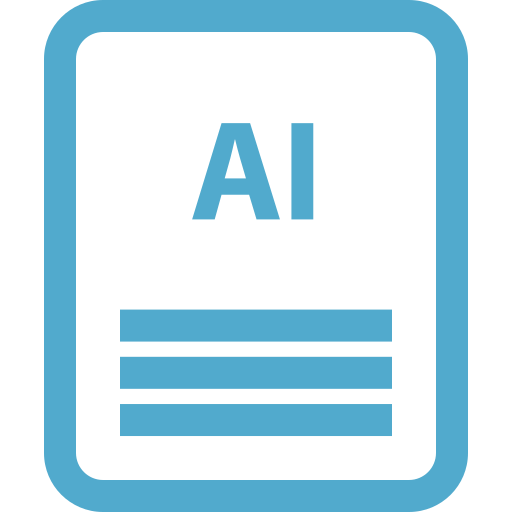
Types of AI: Debunking the mystery
Machine Learning and Deep Learning form the core of Artificial Intelligence
Machine Learning (ML) Often falsely used interchangeably with AI. AI is the umbrella term loosely referring to any application that allows a computer to do something that otherwise requires human intelligence, such as recognizing objects or sounds, understanding natural language, or solving complex problems. Machine learning is a type of AI that enables a computer to learn on its own (without being “programmed”) and improve its task performance over time. Machine Learning focuses on the development of algorithms and models that enable computers to learn from the data and make predictions, and then improve that predictive ability over time.
Most familiar advancements in AI today are the result of machine learning models. Take for example Google Search – once based on rules written by engineers, which limited the number of queries it could handle. Today, powered by machine learning, it can handle billions of queries per day and get better at it over time.
There are different types of machine learning algorithms, but the most common are regression (used to predict outcomes) and classification (used to identify patterns and group data). Machine learning algorithms can be further categorized as supervised (requiring a training data set that includes both the input data and the desired output) and unsupervised (no training dataset, instead relying on the data to “learn” or make the necessary connections on its own.
“Machine Learning focuses on the development of algorithms and models that enable computers to learn from the data and make predictions, and then improve that predictive ability over time.”
Machine Learning Examples
Putting it to use for health care and population health management:
- Machine learning algorithms can speed up prediction of disease development and identification of related risk factors by aggregating and analyzing diverse and often disparate patient data. This may include electronic health records, genetics, lifestyle factors, clinical notes, and input from biometric devices. Detection pathways optimized with machine learning algorithms enable early intervention (much earlier than would have been determined otherwise) and personalized preventive measures informed by a deeper understanding of individual circumstances and health risks.
- For treatment optimization, machine learning algorithms can analyze large stores of data representing patient outcomes, treatment response(s), and adherence to clinical guidelines to determine the most effective treatment options geared toward specific clinical and patient profiles.
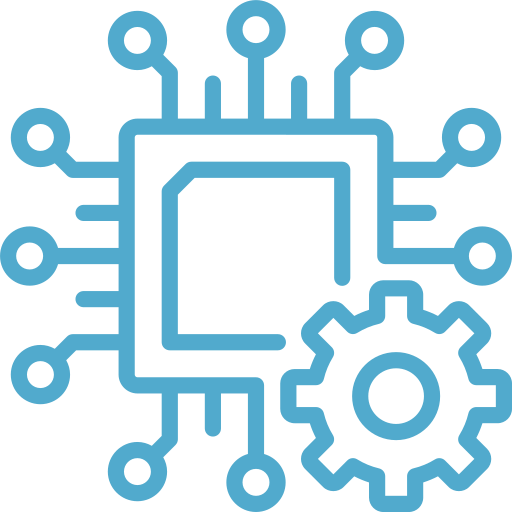
Deep Learning
Deep Learning is considered both a subset of AI and more specifically machine learning. It analyzes data using a tiered algorithmic design, where data is filtered through numerous layers. Each subsequent layer uses output from the preceding one to determine its results. As more data is processed, deep learning models grow more accurate, essentially learning from prior results to improve their capacity to make correlations and connections.
It comprises networks of interconnected processing layers including an “input layer” – input data from the outside, such as an image or sentence, “intermediate layers – where the input layer is processed and passed onto the next layer, and the “output layer” – the result – a prediction or classification, such as identification of a particular object in an image or translation of words from one language to another.
Deep learning networks can perform complex tasks and can be trained to recognize patterns of data that are too complex for humans to identify. Deep learning models can analyze electronic health records that contain structured and unstructured data, including clinical notes, laboratory test results, diagnoses, and medications at exceptional speed and accuracy.
Training is the term used to describe how deep learning networks are taught by continuously adjusting the strength of the connections between each layer – the more data the better the network will be at performing the task it is trained to do. Think of deep learning as a type of machine learning that is used to create models of data, including images, text, and other types of data.
“As more data is processed, deep learning models grow more accurate, essentially learning from prior results to improve their capacity to make correlations and connections.”
Deep Learning Examples
Solving complex health care inquiries
- Pharmaceutical companies are using deep learning applications to identify viable drug combinations by processing genomic, clinical and population data and allowing for insights generated at each “layer” to inform subsequent predictions of efficacy and/or new therapeutic classifications.
- During the height of the Covid-19 pandemic, public and governmental health agencies leveraged deep learning algorithms to estimate benefits of various mitigation measures, and to make predictions of intensive care illness burden, building classification cohorts at high risk of severe disease including need for mechanical ventilation. This is a good example of a public health crisis where time was of the essence, necessitating making the best use of all the pertinent data available at any one time and then continuing to “learn” as new data and information emerged.
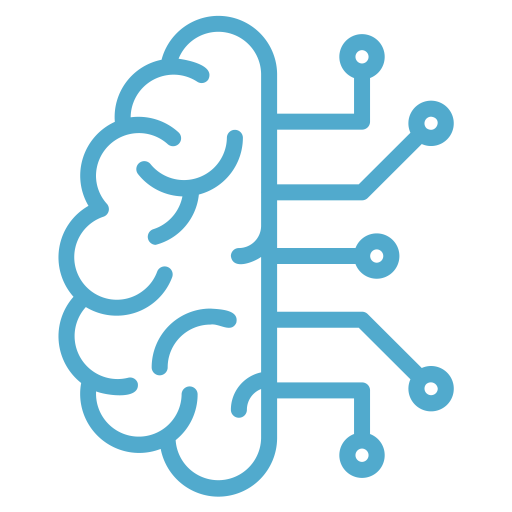
Natural Language Processing (NLP)
NLP deals with the understanding and manipulation of human language. It is a field of AI that has been around for a while but has become more popular of late due to advancements in both Machine and Deep Learning. NLP is used in a variety of applications such as text classification, and machine translation. Google Translate, Siri and Alexa are commonplace examples of applications that use NLP.
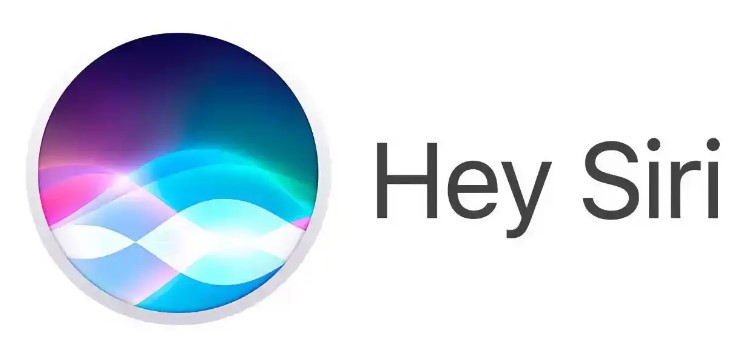
Examples of NLP in health care
Automated transcription of medical records
- NLP algorithm analyzes spoken or written words and converts them into structured electronic formats. This saves time and facilitates efficient retrieval and analysis of patient information.
- NLP can also extract valuable information from unstructured clinical notes, making it easier for healthcare providers to access diagnoses, medications, and treatment plans from vast amounts of textual information. This also enables data aggregation for research, quality improvement and clinical decision support systems.
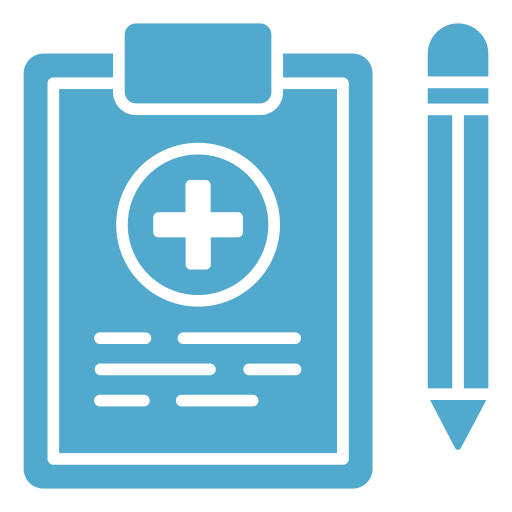
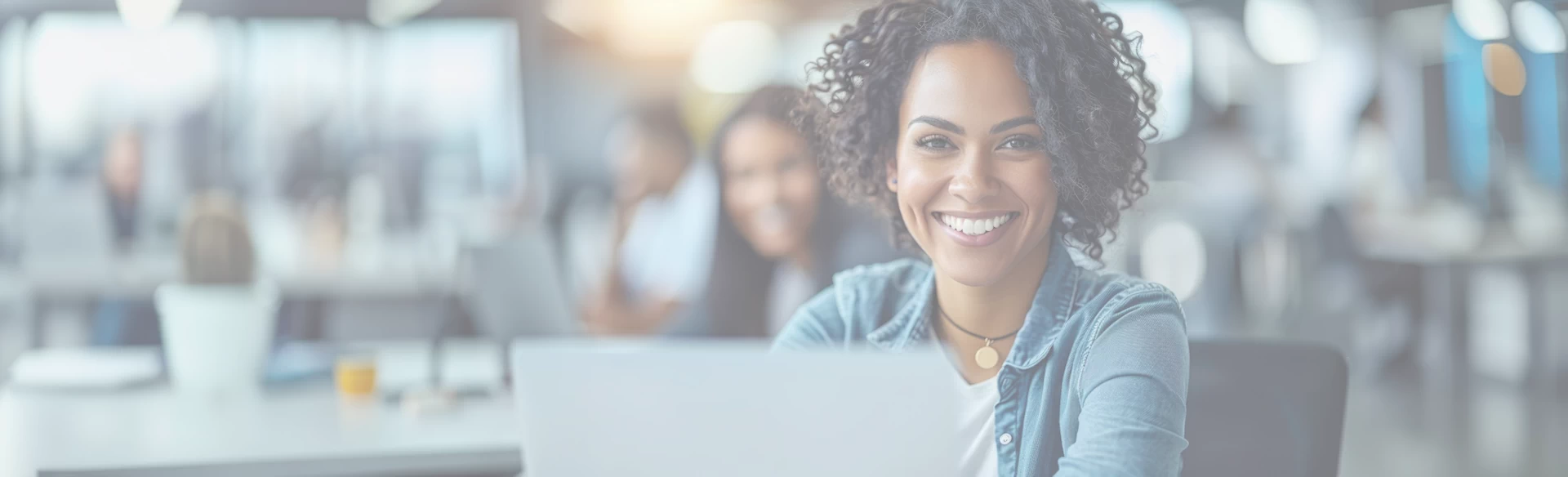
In Summary
As you have read from this brief overview, there are countless practical benefits of AI in healthcare that can help eliminate administrative burdens and improve patient care. By leveraging the power of AI, healthcare providers can achieve higher efficiency, accuracy, and improved patient-centric care.
Looking ahead, AI’s future in healthcare continues to be promising. It has the potential to greatly enhance personalized healthcare delivery, with the ability to integrate and develop algorithms comprising data from wearable devices, electronic health records, and telemedicine platforms.
Health care providers and organizations interested in getting started with AI can begin that journey by focusing on fundamentals such as using AI to offset administrative tasks, improve data quality, and support day to day clinical workflows – doing so with relatively low risk and low upfront investments.
However, integrating AI into healthcare systems requires careful attention to ethical and privacy considerations. Patient data usage must adhere to strict privacy regulations, and biases in AI algorithms need to be scrutinized and mitigated. This and other potential risks need to be weighed against potential benefits, which will require a multifaceted approach that involves transparency, responsible data practices, and robust security measures.
In our next article, we will provide a more in-depth look at what is involved, and related considerations for taking the first steps, as well as some of the specific use cases that can begin to build a foundation for future AI endeavors.
” By leveraging the power of AI, healthcare providers can achieve higher efficiency, accuracy, and improved patient-centric care.”